Group B, Poster #096, Earthquake Geology
Developing a machine learning dataset to facilitate fine-detail post-earthquake fault rupture mapping
Poster Image:
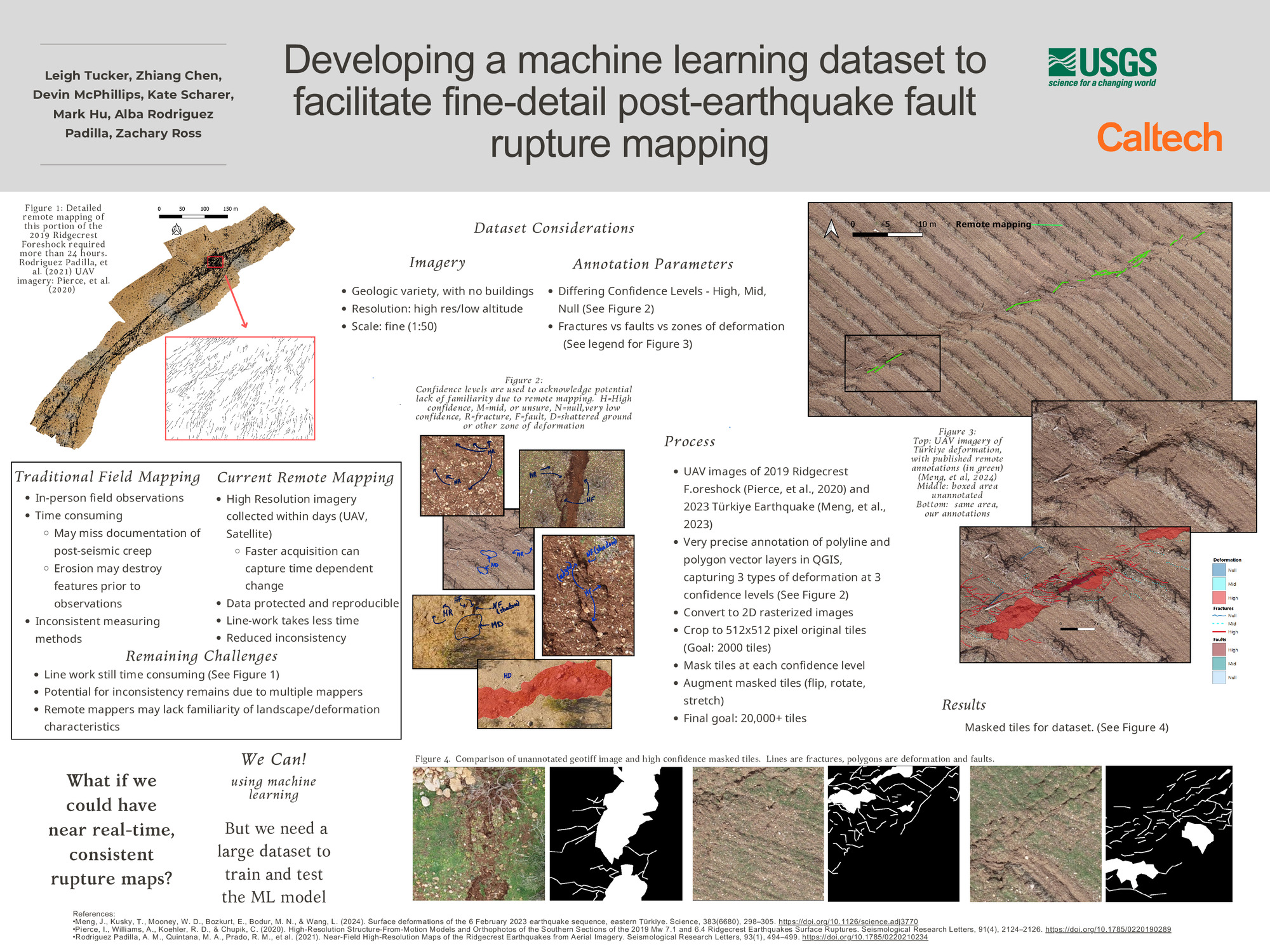
Poster Presentation
2024 SCEC Annual Meeting, Poster #096, SCEC Contribution #13741 VIEW PDF
ning applications in ground rupture and deformation mapping (see accompanying poster by Hu, et al). This dataset is motivated by the understanding that final products could help advance a variety of earthquake science studies. Imagery from earthquakes in California and Türkiye are used to inform the model of multiple surface rupture expressions due to differing geologic settings and land use. To capture a broad range of ground deformation efficiently, we annotate the imagery with both polylines and polygons that define the faults and fault zones, respectively. Annotations are at a scale of approximately 1:50 and across a variety of rupture densities and fault zone widths, providing fine detail while also being able to locate off-fault deformation. The dataset includes annotations at three confidence levels: high, mid, and null. The null confidence level is an important means of informing the model of features such as shadows and plough furrows, which can resemble fractures. Our goal is to have 2000+ unique 512x512 image tiles as a base set which can be increased through augmentation. Future work could include adding annotated imagery from additional locations and/or ruptures through built environments.
SHOW MORE
SHOW MORE