Group A, Poster #005, Seismology
Accelerating Full-Waveform Inversion with Fourier Neural Operators
Poster Image:
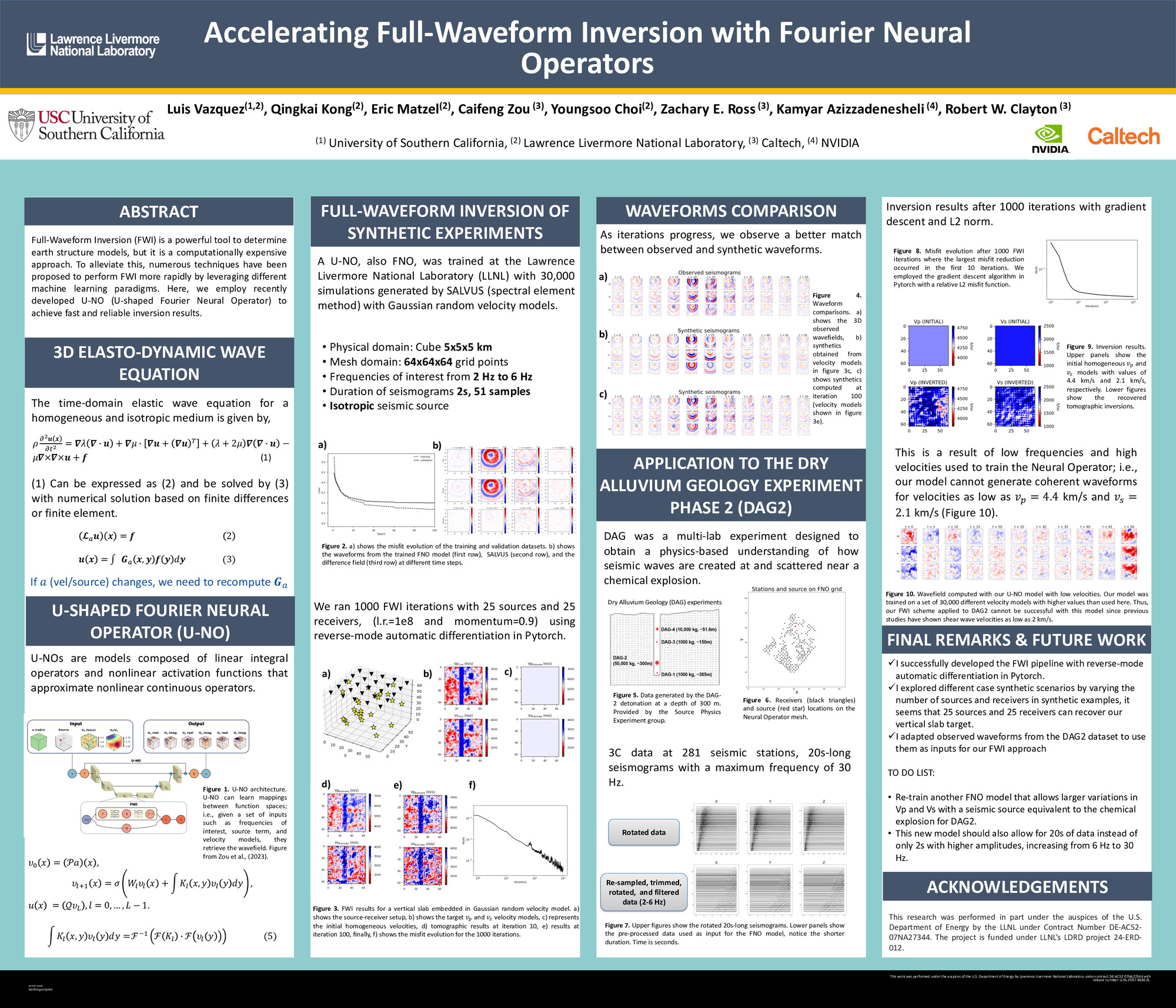
Poster Presentation
2024 SCEC Annual Meeting, Poster #005, SCEC Contribution #13524 VIEW PDF
Full-Waveform Inversion (FWI) is a powerful tool to determine earth structure models, but it is a computationally expensive approach. To alleviate this, numerous techniques have been proposed to perform FWI more rapidly by leveraging different machine learning paradigms. Here, we employ the recently developed U-NO (U-shaped Fourier Neural Operator) to achieve fast inversion.
Previous studies have shown that Fourier Neural Operator based approach can reduce the computational time for FWI by a factor of 350. This work shows the FWI results obtained from a U-NO model trained using the high-performance computing facilities at Lawrence Livermore National Laboratory on a 64x64x64 grid-cube. The model is trained using waveforms from 30,000 different Gaussian random velocity models generated by the spectral-element method. We explore the U-NO model capabilities and limitations with a series of experiments. Our initial results show promising inversions using automatic differentiation of the U-NO model. Finally, we are exploring to implement the workflow to a real dataset, which will be also discussed.
This work was performed under the auspices of the U.S. Department of Energy by Lawrence Livermore National Laboratory under Contract DE-AC52-07NA27344. The document number is LLNL-ABS-XXXXXX.
Previous studies have shown that Fourier Neural Operator based approach can reduce the computational time for FWI by a factor of 350. This work shows the FWI results obtained from a U-NO model trained using the high-performance computing facilities at Lawrence Livermore National Laboratory on a 64x64x64 grid-cube. The model is trained using waveforms from 30,000 different Gaussian random velocity models generated by the spectral-element method. We explore the U-NO model capabilities and limitations with a series of experiments. Our initial results show promising inversions using automatic differentiation of the U-NO model. Finally, we are exploring to implement the workflow to a real dataset, which will be also discussed.
This work was performed under the auspices of the U.S. Department of Energy by Lawrence Livermore National Laboratory under Contract DE-AC52-07NA27344. The document number is LLNL-ABS-XXXXXX.