Group B, Poster #048, Seismology
Comparing the efficacy of deep learning phase pickers and associations for developing earthquake catalogs
Poster Image:
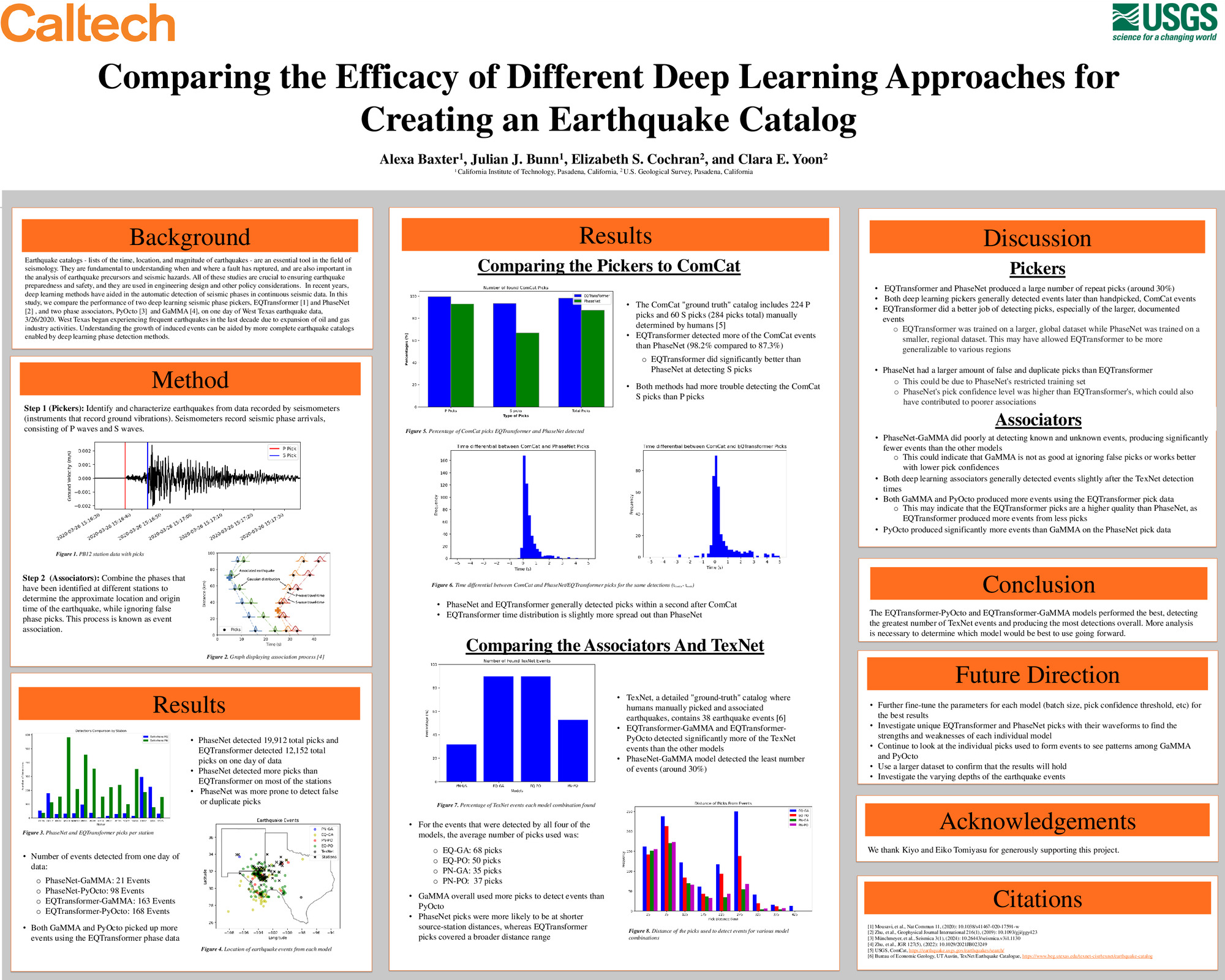
Poster Presentation
2024 SCEC Annual Meeting, Poster #048, SCEC Contribution #13927 VIEW PDF
Earthquake catalogs – lists of the time, location, and magnitude of earthquakes – are an essential tool in the field of seismology for documenting the occurrence and probing the cause and evolution of earthquake sequences. In recent years, deep learning methods have aided in the detection of seismic phases in continuous seismic data. In this study, we compare the performance of two deep learning seismic phase pickers, EQTransformer and PhaseNet, and two phase associators, PyOcto and GaMMA, applied to two days of West Texas earthquake data. West Texas began experiencing frequent induced earthquakes in the last decade due to expansion of oil and gas industry activities. We present preliminary comparisons of the performance of the phase picks produced by EQTransformer and PhaseNet and compare the four event catalogs developed by combining the two sets of phase picks in two associators (EQT-PyOcto, EQT-GaMMA, PhaseNet-PyOcto, PhaseNet-GaMMA). We also compare the phase picks and event detections from our analyses to those contributed by the regional network, TexNet. While catalog events are generally detected, there can be significant variability in the number of phase and event detections across the different catalogs developed. We additionally explore the factors that influence the reliability of the phase pickers and associators applied to this dataset, including station density, source-station distances, or magnitude.