Poster #034, Seismology
EikoNet: Solving the Eikonal equation with Deep Neural Networks, with applications to earthquake location
Poster Image:
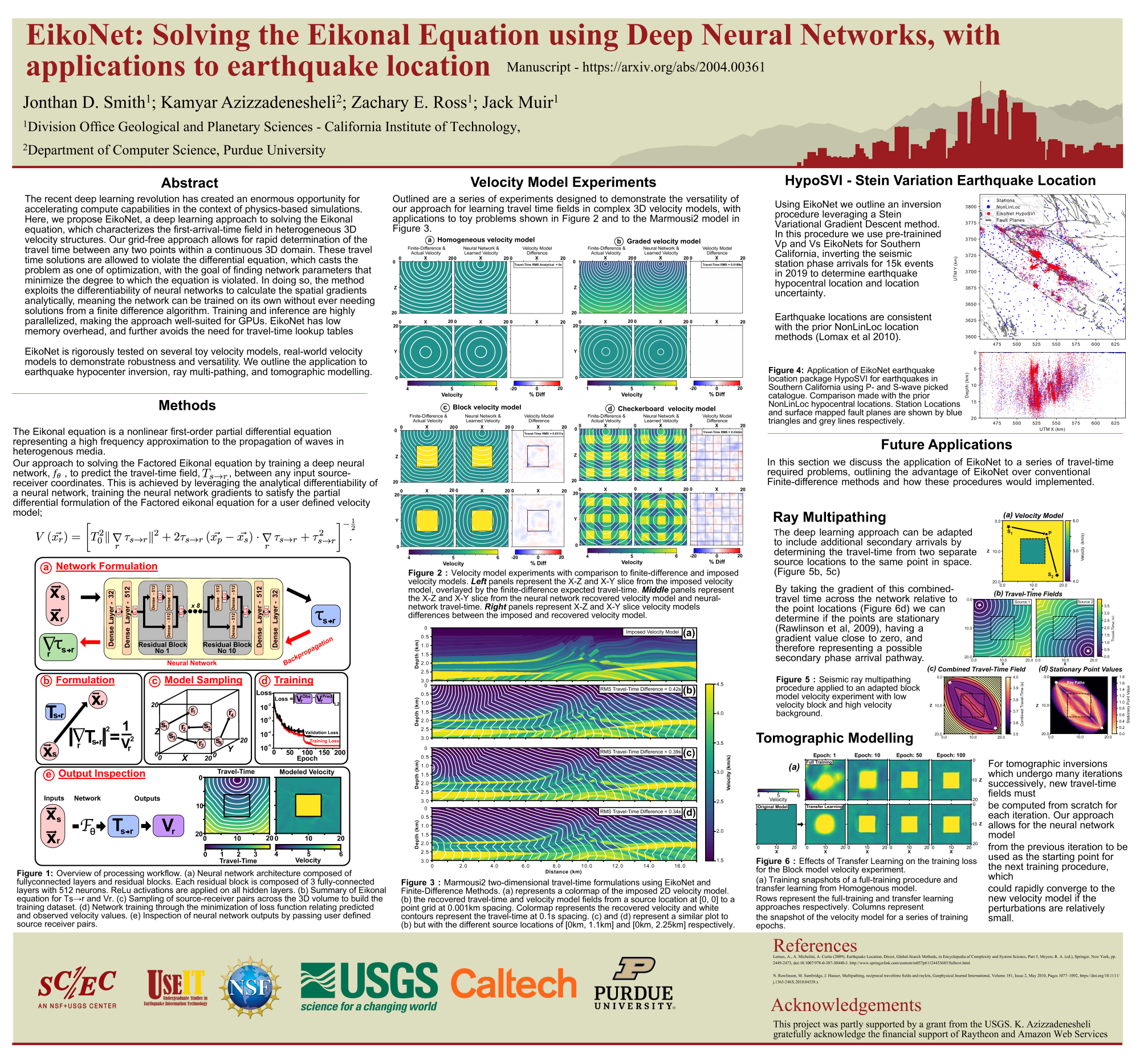
Poster Presentation
2020 SCEC Annual Meeting, Poster #034, SCEC Contribution #10665 VIEW PDF
he method exploits the differentiability of neural networks to calculate the spatial gradients analytically, meaning the network can be trained on its own without ever needing solutions from a finite difference algorithm. Training and inference are highly parallelized, making the approach well-suited for GPUs. EikoNet has low memory overhead, and further avoids the need for travel-time lookup tables
EikoNet is rigorously tested on several toy velocity models, real-world velocity models and sampling methods to demonstrate robustness and versatility. We outlinethe application to earthquake hypocenter inversion, ray multi-pathing, and tomographic modelling. Demonstrating the advantages of this method over conventional finite-difference approaches.
SHOW MORE
EikoNet is rigorously tested on several toy velocity models, real-world velocity models and sampling methods to demonstrate robustness and versatility. We outlinethe application to earthquake hypocenter inversion, ray multi-pathing, and tomographic modelling. Demonstrating the advantages of this method over conventional finite-difference approaches.
SHOW MORE