Group A, Poster #115, Fault and Rupture Mechanics (FARM)
Physics-informed deep learning of rate-and-state fault friction
Poster Image:
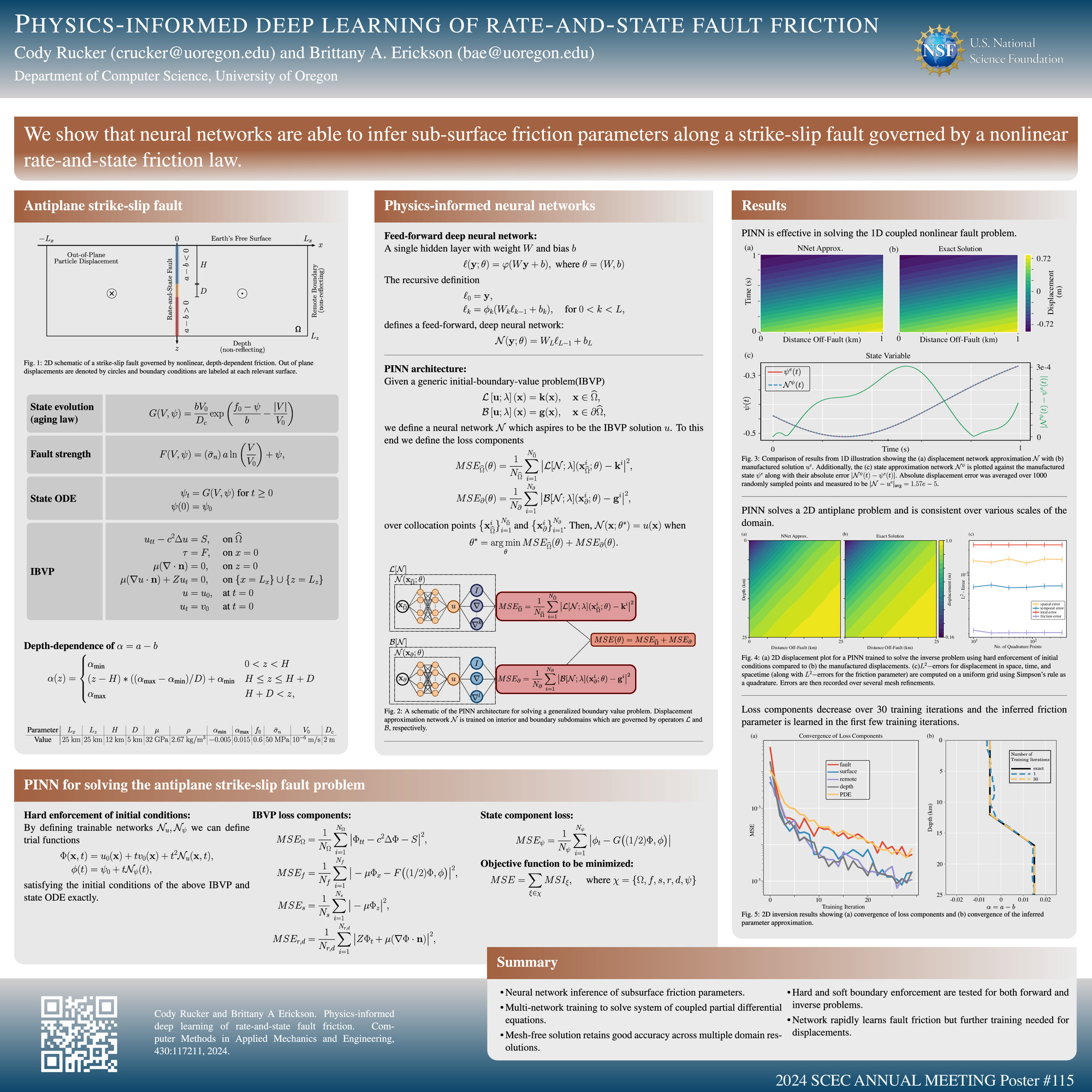
Poster Presentation
2024 SCEC Annual Meeting, Poster #115, SCEC Contribution #13601 VIEW PDF
ions for assessing seismic hazard. We present the computational PINN framework for strike-slip faults in 1D and 2D subject to rate-and-state friction. Initial and boundary conditions define the data on which the PINN is trained. While the PINN is capable of approximating the solution to the governing equations to low-errors, our primary interest lies in the network's capacity to infer friction parameters during the training loop. We find that the network for the parameter inversion at the fault performs much better than the network for material displacements to which it is coupled. Additional training iterations and model tuning resolves this discrepancy, enabling a robust surrogate model for solving both forward and inverse problems relevant to seismic faulting.
SHOW MORE
SHOW MORE