Poster #215, Seismology
Microseismic monitoring using deep learning
Poster Image:
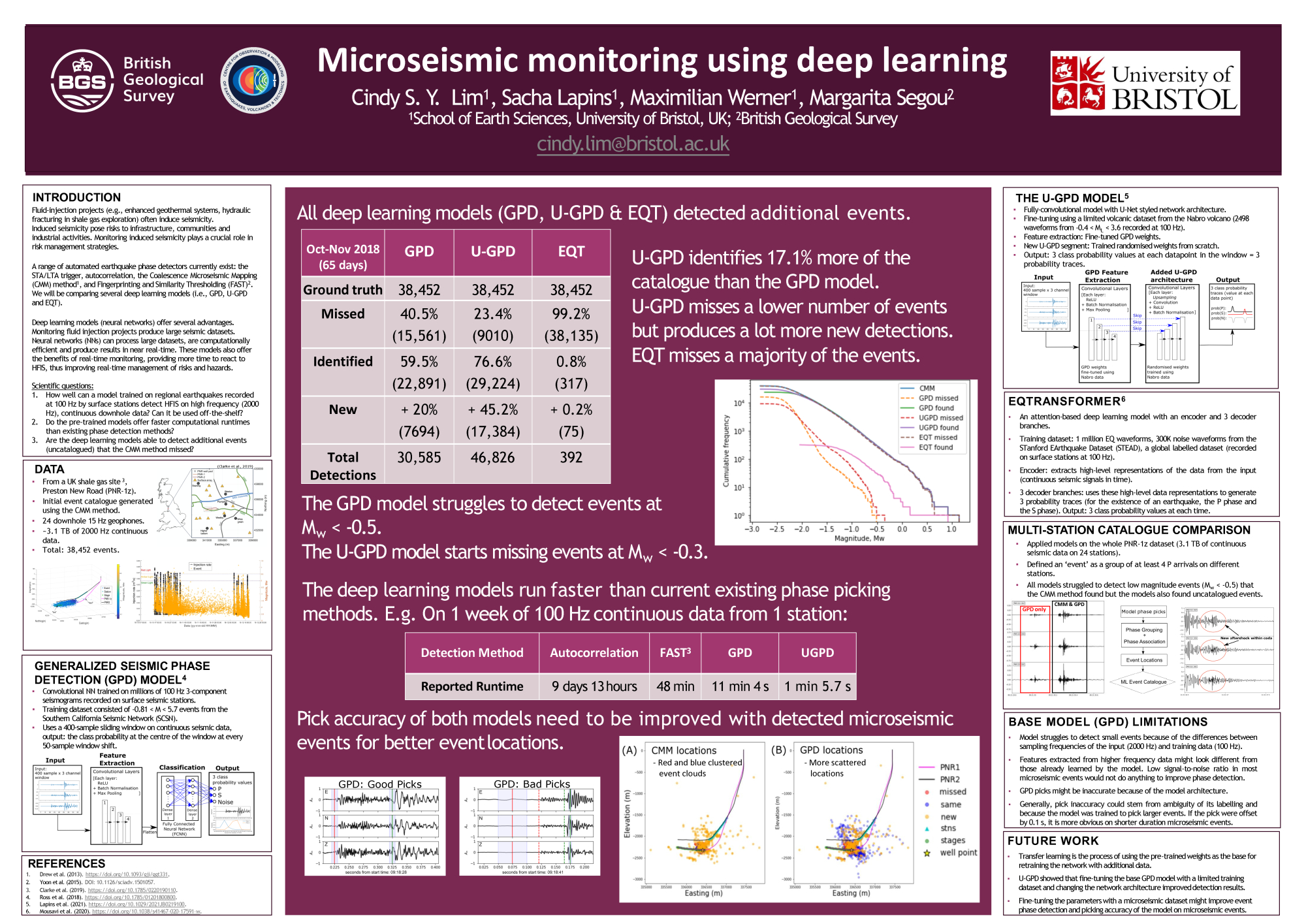
Poster Presentation
2021 SCEC Annual Meeting, Poster #215, SCEC Contribution #11440 VIEW PDF
onal earthquakes. Here, we examine to what extent several prominent pre-trained neural networks can detect HFIS, including the GPD model, EQTransformer, and a more recent U-GPD model. We use data from Preston New Road, a UK shale gas site, where over 38,000 events (-2.8 ≤ Mw ≤ 1.2) were catalogued in 2018 by the coalescence microseismic mapping (CMM) method. Results show that the models managed to identify up to 76.6% (29,224 of 38,452 events) of the pre-existing CMM catalogue and started to struggle with event detection at Mw < -0.5. Although the models miss smaller events, they are able to detect new events previously uncatalogued by the CMM method (e.g., 980 new events within an hour). The models run very efficiently- at least four times faster than existing methods. However, the models do not generally have good picking accuracy and consistent phase detection of microseismic phases (with low signal-to-noise ratio) as they were trained to pick larger, regional phases. This study indicates that pre-trained deep learning models struggle with accurate phase detection of low magnitude, low SNR events; however, they still offer much potential for microseismic monitoring with their efficiency in analysing large datasets.
SHOW MORE
SHOW MORE