Poster #225, Ground Motions
Deep Learning for Site Response Estimation from Geotechnical Array Data
Poster Image:
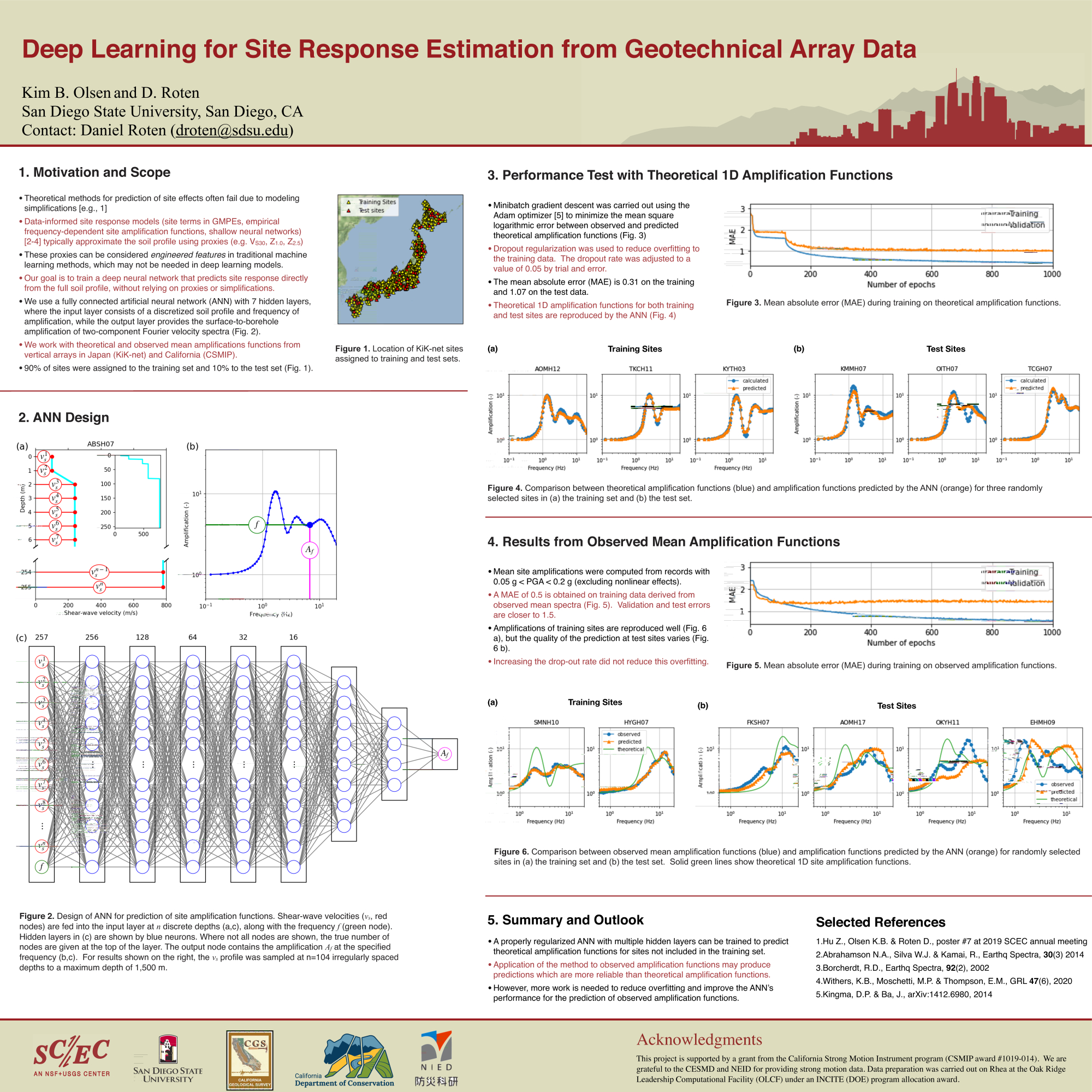
Poster Presentation
2020 SCEC Annual Meeting, Poster #225, SCEC Contribution #10585 VIEW PDF
ency of amplification, as well as earthquake characteristics including magnitude, distance and shaking duration. The output layer consists of a single neuron representing the amplification at the selected frequency. Experiments with 600 real KiK-net soil profiles show that a properly regularized ANN with seven hidden layers is able to learn the theoretical SH amplification functions, and predict the site response for profiles that were not part of the training set. We train the network using a large number of observed amplifications from vertical arrays located in California (CSMIP) and Japan (KiK-net). We find that predicting observed transfer functions from unsmoothed velocity profiles requires more hidden layers than predicting theoretical amplifications from simplified soil profiles. We need at least nine hidden layers to obtain low bias while training the network with transfer functions observed at Japanese and Californian vertical arrays.
We experiment with different network architectures and regularization strategies to improve the predictions and to avoid overfitting. The accuracy of the network is evaluated against traditional (theoretical) site response assessment techniques for randomly chosen sites and earthquakes which were not part of the training dataset.
SHOW MORE
We experiment with different network architectures and regularization strategies to improve the predictions and to avoid overfitting. The accuracy of the network is evaluated against traditional (theoretical) site response assessment techniques for randomly chosen sites and earthquakes which were not part of the training dataset.
SHOW MORE