Poster #211, Ground Motions
Ground-Motion Time-Series Interpolation within the Community Seismic Network using Gaussian Process Regression: Application to the 2019 Ridgecrest Earthquake
Poster Image:
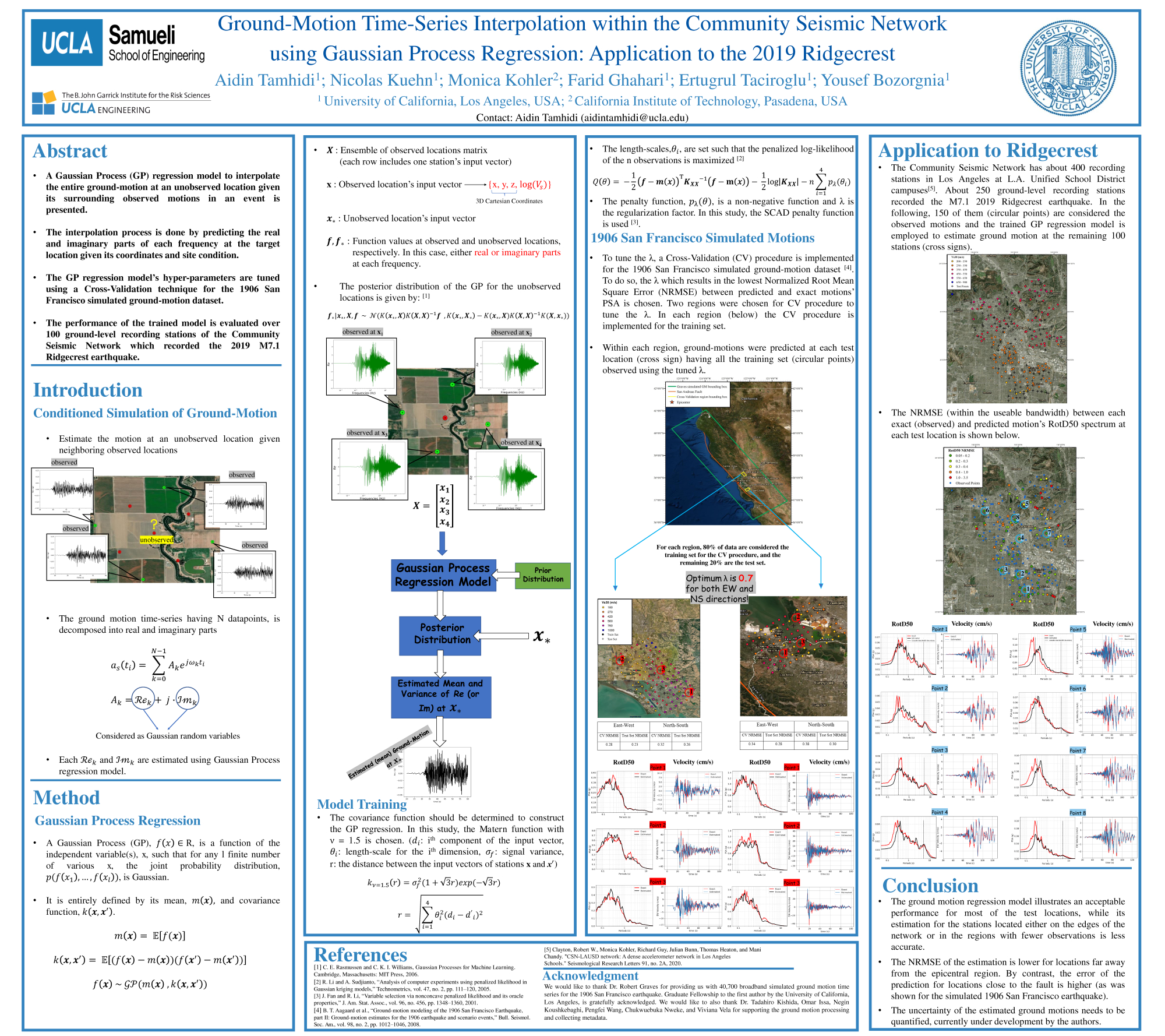
Poster Presentation
2020 SCEC Annual Meeting, Poster #211, SCEC Contribution #10623 VIEW PDF
ls. However, the estimated GMIMs are not adequate if nonlinear time history analysis of structures at uninstrumented locations is required. Therefore, the estimation of the entire ground-motion time series is crucial in order to recognize the dynamic responses of the structures. In addition, one can have a better damage and loss estimation by estimating the entire ground motion time series at various uninstrumented locations after an earthquake, which will provide the responsible agencies with an accurate post-earthquake assessment. In this study, an interpolation method to predict the entire ground motion time series from neighboring stations’ recorded motions is presented. The interpolation process is done using a Gaussian Process regression, which models the real and imaginary parts for various frequencies as random Gaussian variables. The Gaussian Process regression model eventually estimates the entire ground motion time series, given the geographic coordinates and site conditions of the target locations. The training and verification of the proposed model are carried out using physics-based simulated ground motions of the 1906 San Francisco earthquake. Eventually, the 257 ground-level recording stations of the Community Seismic Network that recorded the 2019 Ridgecrest earthquake within Los Angeles are employed to test and evaluate the performance of the Gaussian Process regression model.
SHOW MORE
SHOW MORE