Group A, Poster #195, Earthquake Forecasting and Predictability (EFP)
Forecasting the 2016-2017 Central Apennines Earthquake Sequence with a Neural Marked Point Process
Poster Image:
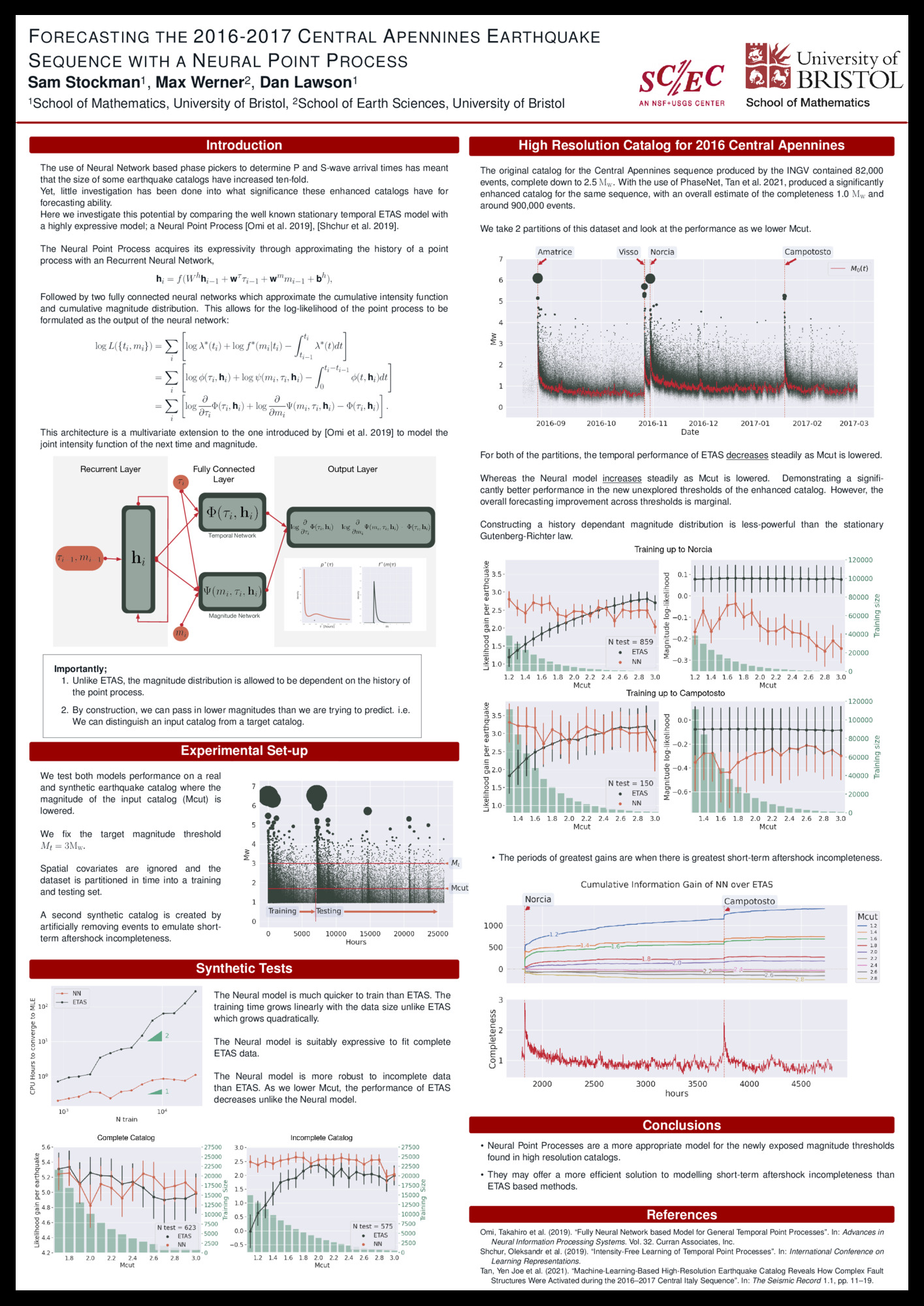
Poster Presentation
2022 SCEC Annual Meeting, Poster #195, SCEC Contribution #11932 VIEW PDF
Point processes have been the dominant way of modeling the evolution of seismicity for decades, with the Epidemic Type Aftershock Sequence (ETAS) model being the most popular. Recent advances in the field of machine learning have constructed highly flexible point process models using neural networks to seek improvements on existing parametric models. We investigate whether these flexible point process models can be applied to short term seismicity forecasting by extending an existing temporal neural model to the magnitude domain and we show how this model can be used to forecast earthquakes above a target magnitude threshold. We first demonstrate that the neural model can learn to fit synthetic ETAS data. However, this requires less computational time due to the fact that it is not dependent on the full history of the sequence. On new high-resolution data from an earthquake sequence in the Central Apennines 2016-2017, we investigate the sensitivity of both ETAS and the neural model with respect to the lowest magnitude passed into each. We find that both models perform similarly at previously explored thresholds but as the magnitude is lowered the performance of ETAS reduces unlike that of the neural model. We argue that some of the gains made by the neural model are due to its ability to handle incomplete data. Since neither model has an overall forecasting advantage over the other, our work suggests that there is not enough signal in 2-dimensional data to give a neural model an advantage over ETAS, but does offer a more efficient way to model data incompleteness. Our work also stresses the need for considering temporal incompleteness when using new machine learning generated earthquake catalogs.