Group B, Poster #222, Research Computing (RC)
Challenges of incorporating Machine Learning and DAS into the Southern California Seismic Network (SCSN) and Southern California Earthquake Data Center (SCEDC)
Poster Image:
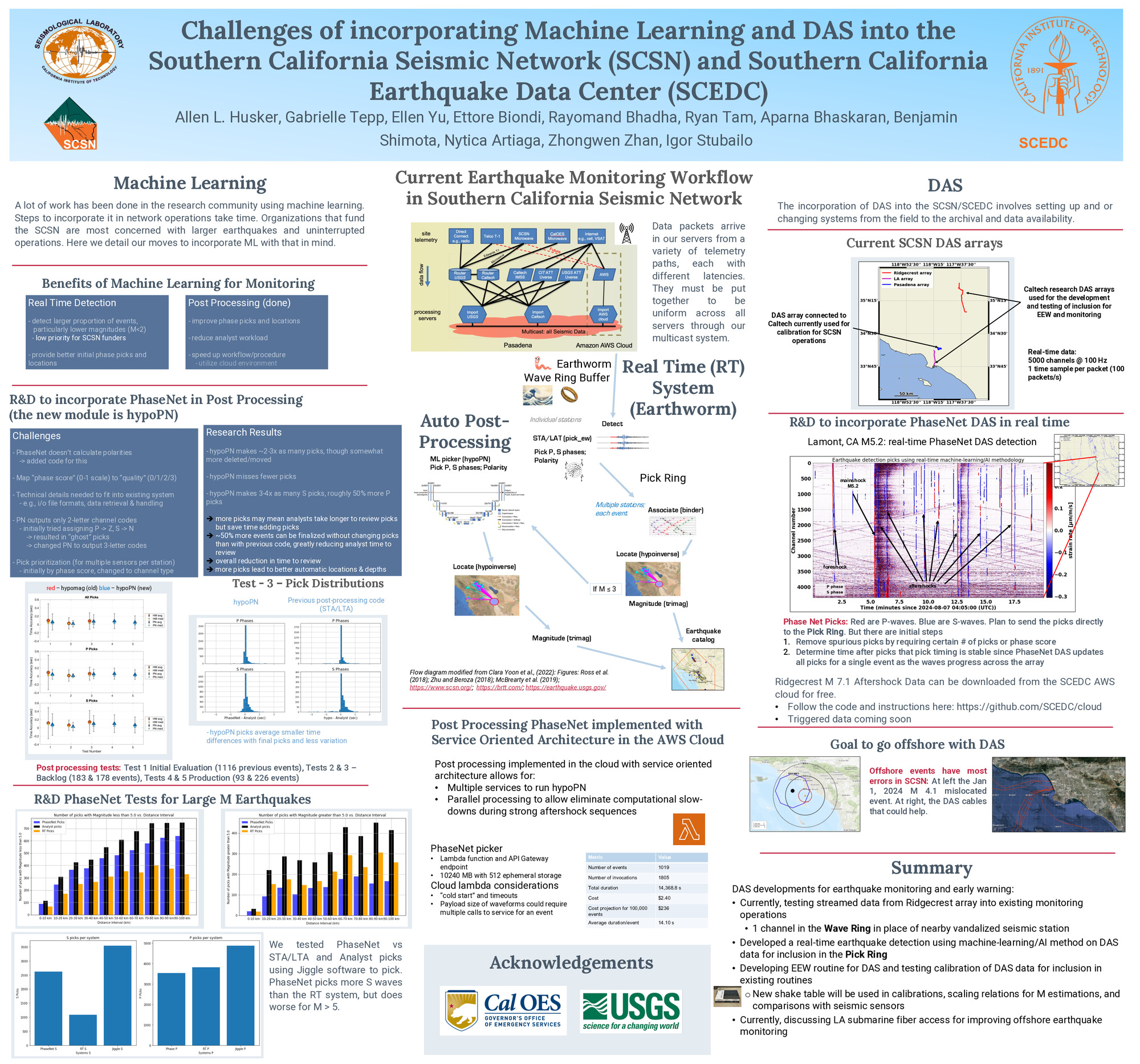
Poster Presentation
2024 SCEC Annual Meeting, Poster #222, SCEC Contribution #14001 VIEW PDF
research purposes and often overlook factors important for real-time monitoring, such as processing time, computational requirements, and data delays and incompleteness. Moreover, our RT system, Earthworm/AQMS, is now very old and was not written in a way to easily test or change individual parts. We therefore are using a 3-pronged approach to incorporate ML: 1) use ML in postprocessing to get the benefits of additional and more accurate picks for already-detected earthquakes, 2) provide RT data files to replay so that researchers can train ML codes on it, and 3) incorporate ML into the RT system by revising code to meet requirements of operation. Tests of the ML picker show that it outperforms the RT system only for earthquakes M<5.
DAS can be incorporated in the RT system in several ways that are currently being tested or already in use. The Ridgecrest DAS array is sending 5000 channels at 100 sps every second to the SCSN. We plan to incorporate 18 of these channels into our earthquake detection process. We have been acquiring DAS in real-time for many months, and the system keeps up with a feed of 5000 channels. The next step is to send 1 channel into the RT system as the equivalent of a seismic station to test real-time DAS in our system. However, the best option for earthquake monitoring is to use the benefits of the entire DAS array. We recently succeeded in using the ML picker PhaseNet-DAS for real-time phase picking. Eventually, we plan to submit picks directly into the pick-ring that goes to the associator and earthquake locator. The final steps for the SDEDC are to archive DAS data including station metadata. DAS data is large and will be saved as triggered data per event. Then it will be made accessible through similar methods used with seismic data through the cloud.
SHOW MORE
DAS can be incorporated in the RT system in several ways that are currently being tested or already in use. The Ridgecrest DAS array is sending 5000 channels at 100 sps every second to the SCSN. We plan to incorporate 18 of these channels into our earthquake detection process. We have been acquiring DAS in real-time for many months, and the system keeps up with a feed of 5000 channels. The next step is to send 1 channel into the RT system as the equivalent of a seismic station to test real-time DAS in our system. However, the best option for earthquake monitoring is to use the benefits of the entire DAS array. We recently succeeded in using the ML picker PhaseNet-DAS for real-time phase picking. Eventually, we plan to submit picks directly into the pick-ring that goes to the associator and earthquake locator. The final steps for the SDEDC are to archive DAS data including station metadata. DAS data is large and will be saved as triggered data per event. Then it will be made accessible through similar methods used with seismic data through the cloud.
SHOW MORE