Group B, Poster #224, Ground Motions
Reduced-order modeling for physics-based ground motion simulation
Poster Image:
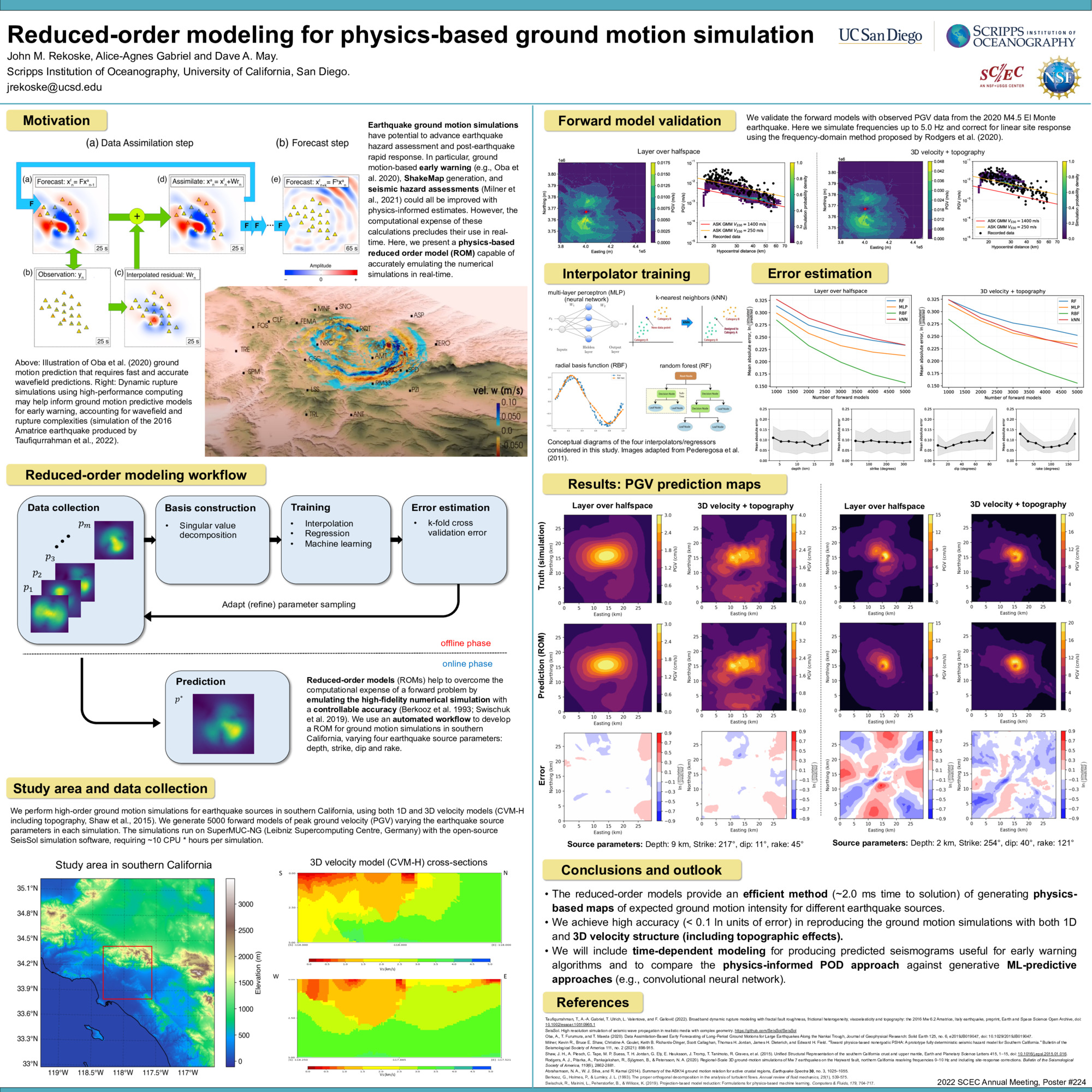
Poster Presentation
2022 SCEC Annual Meeting, Poster #224, SCEC Contribution #12224 VIEW PDF
ient training data to fully train certain ML algorithms. The complementary strengths of ML and physics-based modeling motivates new research to develop hybrid models that combine the two.
To that end, here we explore the applicability of combining data-driven reduced order models (ROMs) with physics-based forward simulations of ground motion to predict peak ground velocity (PGV) maps. Specifically, we evaluate the interpolated proper orthogonal decomposition-based ROM by considering the southern California region and simulating synthetic earthquakes in which the focal mechanism and depth are taken as the model parameters. We explore the ROM accuracy when using radial basis functions, neural networks, k-nearest neighbors, and decision tree interpolators, and when either a 1D or 3D subsurface velocity model is employed. We validate this reduced-order modeling approach with recorded PGV data from the M4.5 2020 South El Monte earthquake. We find that the reduced-order models accurately predict the recorded PGV data, and we compare this prediction accuracy against NGA-West2 ground motion model predictions. We envision that these ROMs will be useful for real-time ShakeMap generation and EEW algorithms that could benefit from physics-based ground motion prediction.
SHOW MORE
To that end, here we explore the applicability of combining data-driven reduced order models (ROMs) with physics-based forward simulations of ground motion to predict peak ground velocity (PGV) maps. Specifically, we evaluate the interpolated proper orthogonal decomposition-based ROM by considering the southern California region and simulating synthetic earthquakes in which the focal mechanism and depth are taken as the model parameters. We explore the ROM accuracy when using radial basis functions, neural networks, k-nearest neighbors, and decision tree interpolators, and when either a 1D or 3D subsurface velocity model is employed. We validate this reduced-order modeling approach with recorded PGV data from the M4.5 2020 South El Monte earthquake. We find that the reduced-order models accurately predict the recorded PGV data, and we compare this prediction accuracy against NGA-West2 ground motion model predictions. We envision that these ROMs will be useful for real-time ShakeMap generation and EEW algorithms that could benefit from physics-based ground motion prediction.
SHOW MORE