Group B, Poster #094, Earthquake Geology
Advancing deep learning approaches to facilitate rapid post-earthquake fault mapping
Poster Image:
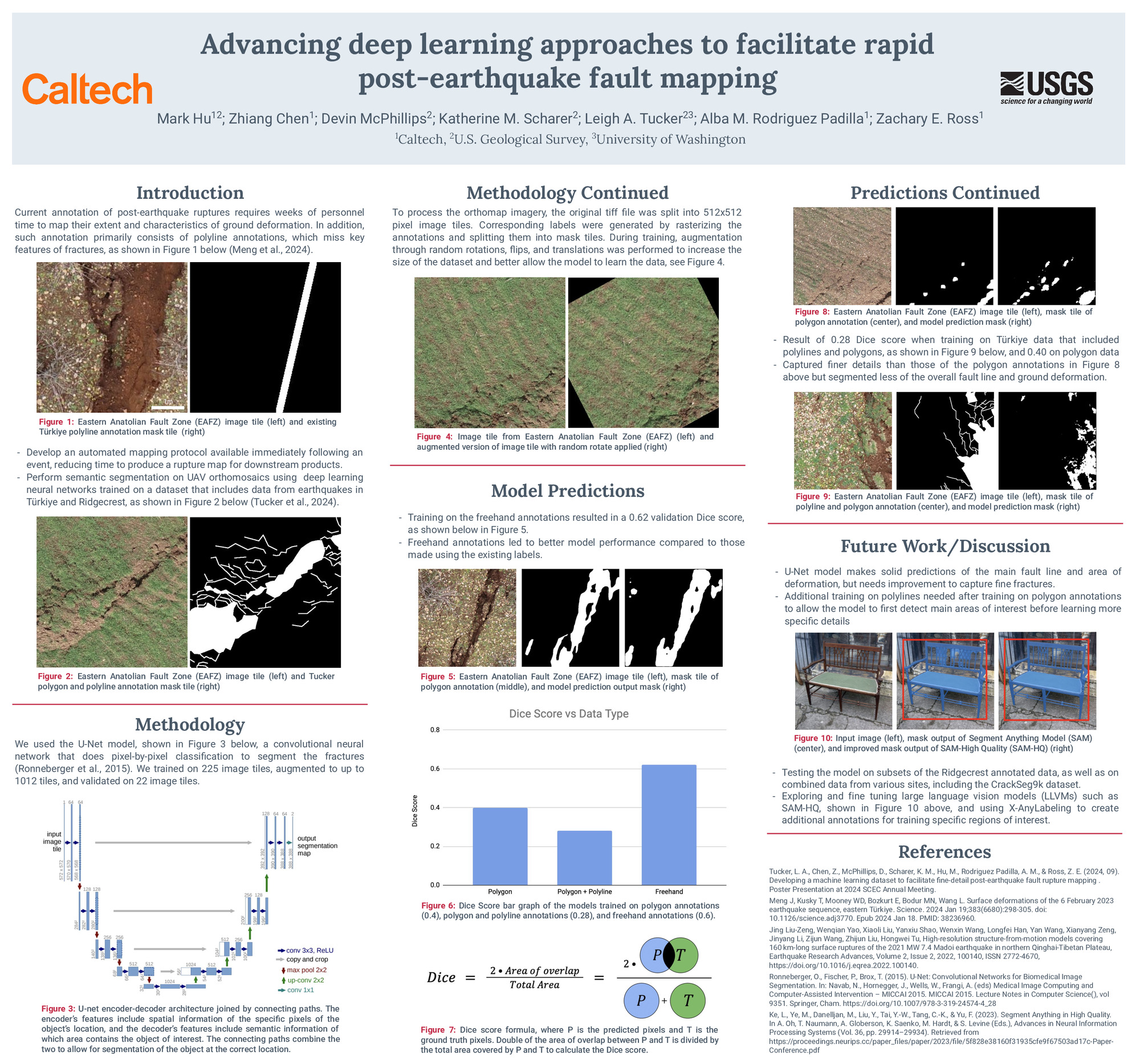
Poster Presentation
2024 SCEC Annual Meeting, Poster #094, SCEC Contribution #13738 VIEW PDF
s to automatically identify and extract characteristics of the rupture and surface deformation. To accomplish this process, deep learning neural networks perform semantic segmentation on high-resolution UAV orthomosaics, capturing the details of the surface fractures. Using a combined dataset that includes data from earthquakes in Türkiye, China, and California (see accompanying poster by Tucker et al.), as well as bedrock data and crack data from CrackSeg9k, we train the UNet architecture model to segment the fractures. (In image processing, segmentation refers to the process of labeling pixels for subsequent analysis.) Results of the model indicate good mapping of the major fault trace on both training, validation, and test datasets. We additionally provide examples of neural network results, post-processed by edge detection, aiming to capture fine-scale features. Future work will extract fracture orientation, density, and width from the model results.
SHOW MORE
SHOW MORE