Group A, Poster #013, Seismology
Improvements to a Graph Neural Network Earthquake Phase Associator
Poster Image:
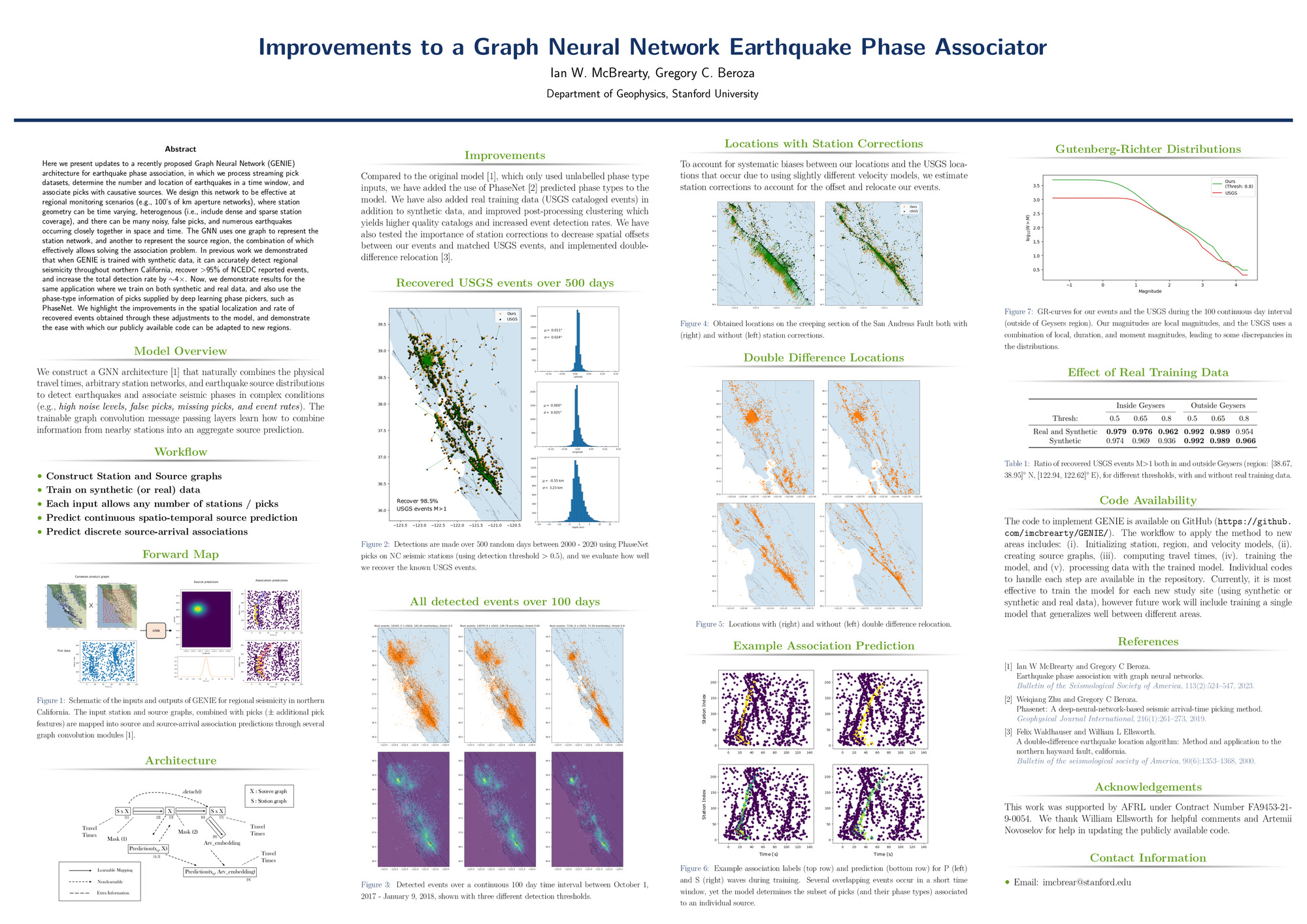
Poster Presentation
2023 SCEC Annual Meeting, Poster #013, SCEC Contribution #13213 VIEW PDF
Here we present updates to a recently proposed Graph Neural Network (GENIE) architecture for earthquake phase association, in which we process streaming pick datasets, determine the number and location of earthquakes in a time window, and associate picks with causative sources. We design this network to be effective at regional monitoring scenarios (e.g., 100’s of km aperture networks), where station geometry can be time varying, heterogenous (i.e., include dense and sparse station coverage), and there can be many noisy, false picks, and numerous earthquakes occurring closely together in space and time. The GNN uses one graph to represent the station network, and another to represent the source region, the combination of which effectively allows solving the association problem. In previous work we demonstrated that when GENIE is trained with synthetic data, it can accurately detect regional seismicity throughout northern California, recover >95% of NCEDC reported events, and increase the total detection rate by ~4x. Now, we demonstrate results for the same application where we train on both synthetic and real data, and also use the phase-type information of picks supplied by deep learning phase pickers, such as PhaseNet. We highlight the improvements in the spatial localization and rate of recovered events obtained through these adjustments to the model, and demonstrate the ease with which our publicly available code can be adapted to new regions.