Group B, Poster #122, Computational Science (CS)
Neural Implicit Compact Representation to Compress Distributed Acoustic Sensing Data
Poster Image:
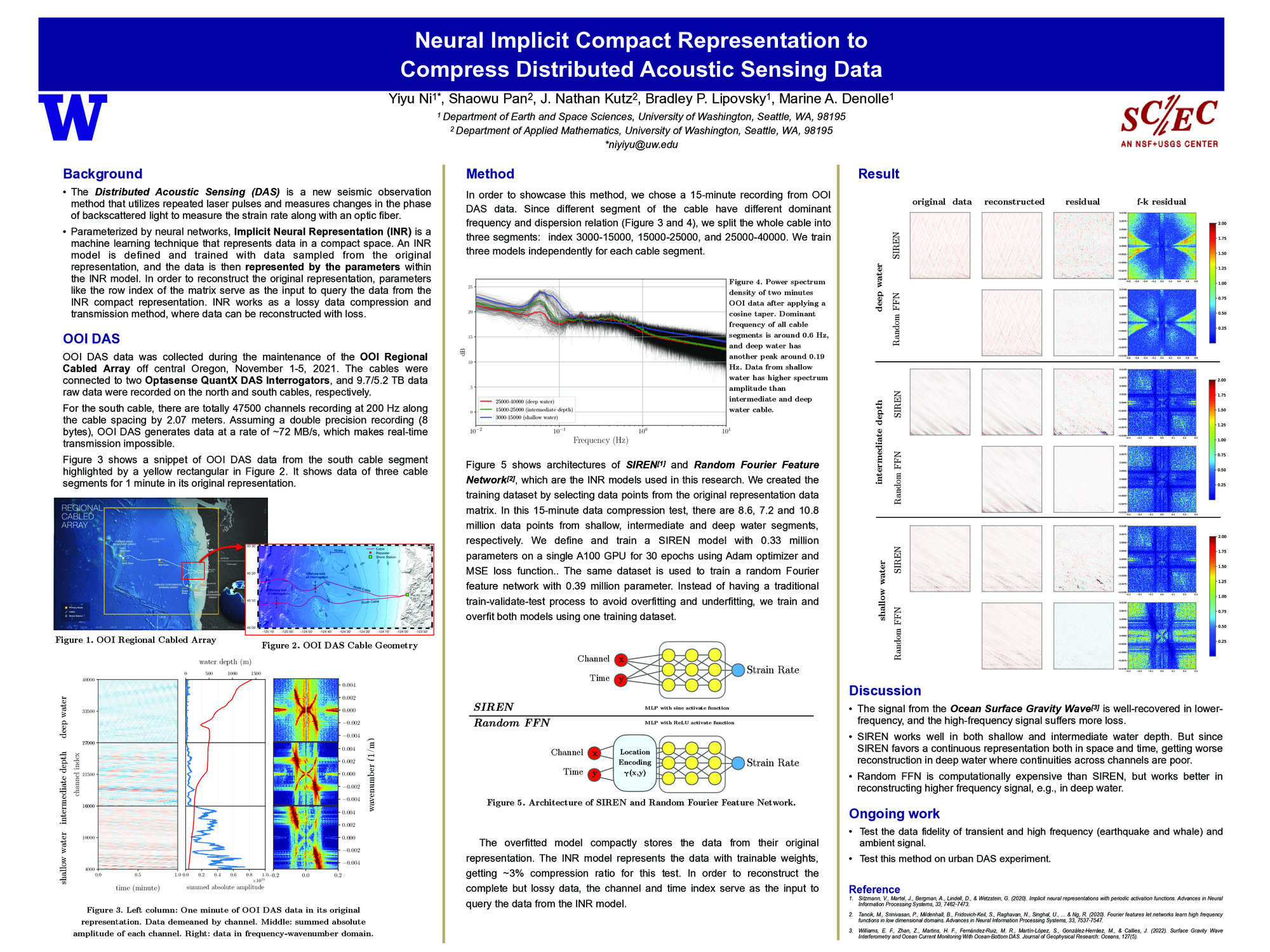
Poster Presentation
2022 SCEC Annual Meeting, Poster #122, SCEC Contribution #12575 VIEW PDF
Distributed Acoustic Sensing (DAS) is a new seismic observation method. It utilizes repeated laser pulses along optical fibers up to 100 km in length to measure changes in phase changes of backscattered light that occur due to rapid straining rate of the fiber. DAS dramatically expands the ability of dense seismic observation and has been used for detecting new tectonic faults and near-surface imaging. However, the high cost of both data transmission and storage limits the real-time or large-scale application of DAS that usually generates terabytes of data per week. Current state-of-the-art data compression techniques for DAS involve either a low compression rate (40%) for a lossless compression (Dong et al., 2022) or lossy compressions that retain the low rank representation of the data.
Here, we use a neural implicit compact representation method to compress an off-shore DAS data set. We used the data from a 4-day ocean-bottom DAS experiment on the Ocean Observatory Initiative (OOI) Regional Cabled Array to test this approach and achieved a lossy compression ratio on the order of 0.1%. We test the fidelity of the reconstructed data on both the residual and specific seismological use cases: the extraction of dispersion curves in the frequency-wavenumber domains, the cross-correlation between and at single channels for ambient-noise imaging and monitoring. This lossy compression-reconstruction approach also showcases a new framework for the real-time application of the DAS data.
Here, we use a neural implicit compact representation method to compress an off-shore DAS data set. We used the data from a 4-day ocean-bottom DAS experiment on the Ocean Observatory Initiative (OOI) Regional Cabled Array to test this approach and achieved a lossy compression ratio on the order of 0.1%. We test the fidelity of the reconstructed data on both the residual and specific seismological use cases: the extraction of dispersion curves in the frequency-wavenumber domains, the cross-correlation between and at single channels for ambient-noise imaging and monitoring. This lossy compression-reconstruction approach also showcases a new framework for the real-time application of the DAS data.